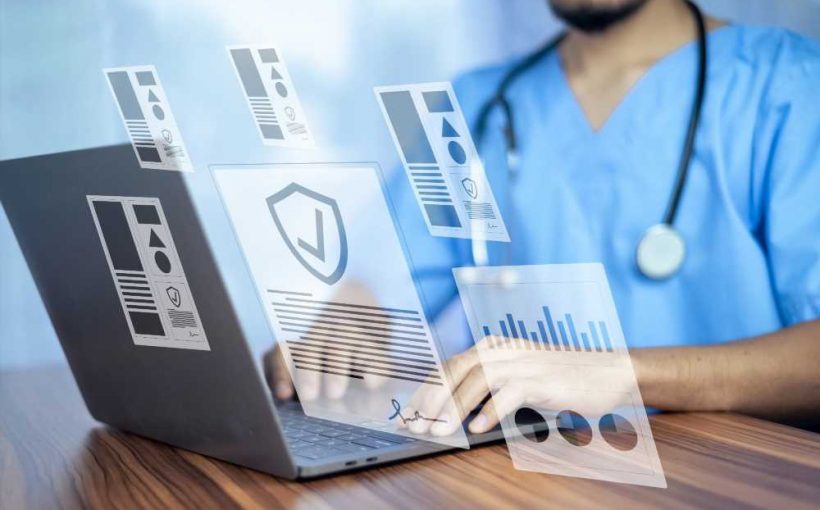
Optimizing hospital patient safety: Machine learning model enhances early warning system performance
In a recent article published in eClinicalMedicine, researchers propose a novel predictive model based on machine learning (ML) for the early prediction of adverse events (AEs), such as cardiac arrest and death, in hospitalized patients using retrospectively collected deterioration index (DI) scores. The performance of this tool was compared with the currently deployed proprietary early warning systems (EWSs) utilizing the DI exceeding 60 hypothesis used to predict a composite AE that includes cardiac arrest, all-cause mortality, and need for an intensive care unit (ICU) admission.
Study: Novel machine learning model to improve performance of an early warning system in hospitalized patients: a retrospective multisite cross-validation study. Image Credit: MUNGKHOOD STUDIO / Shutterstock.com
Background
In the current study, researchers thoroughly searched PubMed for published papers in any language from database inception until September 28, 2023, using keywords including “artificial intelligence (AI)” OR “machine learning” AND “deterioration index,” which led to 454 results. However, none of the identified studies used an ML-based tool for the early prediction of AEs using DI scores.
Currently, most United States hospitals use the Epic DI (EDI) to stratify risk among hospitalized patients and regularly update this index at 15-minute intervals until discharge. Several parameters are considered in the calculation of DI scores, including age, oxygen requirement, and vital sign measurements, as well as routinely recorded physiological, laboratory, and clinical parameters.
EWSs used in the U.S. allowed doctors during the coronavirus disease 2019 (COVID-19) pandemic to intervene early in hospitalized patients at an increased risk of AEs. More specifically, DI scores ranging from low (less than 30), intermediate (30-60), and high (over 60) reflected the risk of a composite AE.
Since accurate detection of deteriorating health before any AE is essential to prevent morbidity and mortality in hospital settings, researchers speculate that ML algorithms incorporating threshold-based EWS or DI scores might perform better in hospitalized patients. However, skepticism persists among clinicians due to methodological weakness, appropriate outcomes, and lack of evidence of its effectiveness after implementation.
About the study
In the present study, researchers used retrospectively collected DI scores for adult hospitalized patients admitted to four Mayo Clinics in the U.S. for medical services between August 23, 2021, and March 31, 2022. In the U.S., the Mayo Clinic provides healthcare services at different geographical sites and maintains integrated electronic health records (EHR) across all locations.
The collected DI scores were represented in a high-dimensional (HD) space using random convolution kernels to help train classifiers (ML models) and calculate the area under the receiver operator characteristics curve (AUC). These predictive tools then analyzed several time intervals before the onset of an AE.
This model was subsequently tested on a previously trained retrospective cohort of hospital encounters. Notably, HD representations significantly improve the discriminative power of ML models, including time series classification and accuracy.
A leave-one-out cross-validation protocol was also used to evaluate the models' performance across each clinical Mayo site.
Study findings
Of the three classifier algorithms, XGBoost trained with the HD features had the best 10-fold cross-validated accuracy with a mean of 0.88, sensitivity and specificity of 0.85 and 0.91, respectively, and F1-score of 0.88.
The accuracy of the other two models, Ridge and SVM, as revealed by their AUCs was 0.85 and 0.76, respectively, while that of the best model XBoost was 0.94. The time interval analysis indicated that XGBoost provided acceptable performance over a 12-hour prediction window. Multisite cross-validation further confirmed the broad applicability of XGBoost across four geographically distinct clinical sites with heterogeneous patient populations.
The innovation of the study model is that it used the entire series of DI scores, rather than a single DI score used in the threshold approach, which significantly improved its predictive potential. Furthermore, this new model compared favorably with five commonly used EWSs. For example, the National Early Warning Score (NEWS) had an AUC of 0.87 based on published literature but 0.94 as compared with the study model.
Conclusions
The current study presents a novel ML algorithm for the early prediction of AEs in hospitalized patients using the entire series of their Epic DI scores. Moreover, this model delivered high classification performance across a broad spectrum of ML tasks, especially the XGBoost classifier.
XBoost also performed better at outcome prediction than the currently used threshold model. Furthermore, its successful multisite cross-validation demonstrated the feasibility of its clinical implementation.
The study findings provide evidence for the cost-effectiveness and high accuracy of this technology, thus supporting its future incorporation in clinical settings.
- Salehinejad, H., Meehan, A. M., Rahman, P. A., et al. (2023). Novel machine learning model to improve performance of an early warning system in hospitalized patients: a retrospective multisite cross-validation study. eClinicalMedicine doi:10.1016/j.eclinm.2023.102312
Posted in: Device / Technology News | Medical Science News | Medical Research News | Healthcare News
Tags: Artificial Intelligence, Cardiac Arrest, Coronavirus, covid-19, Healthcare, Hospital, Intensive Care, Laboratory, Language, Machine Learning, Mortality, Oxygen, Pandemic, Technology
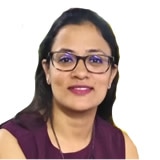
Written by
Neha Mathur
Neha is a digital marketing professional based in Gurugram, India. She has a Master’s degree from the University of Rajasthan with a specialization in Biotechnology in 2008. She has experience in pre-clinical research as part of her research project in The Department of Toxicology at the prestigious Central Drug Research Institute (CDRI), Lucknow, India. She also holds a certification in C++ programming.